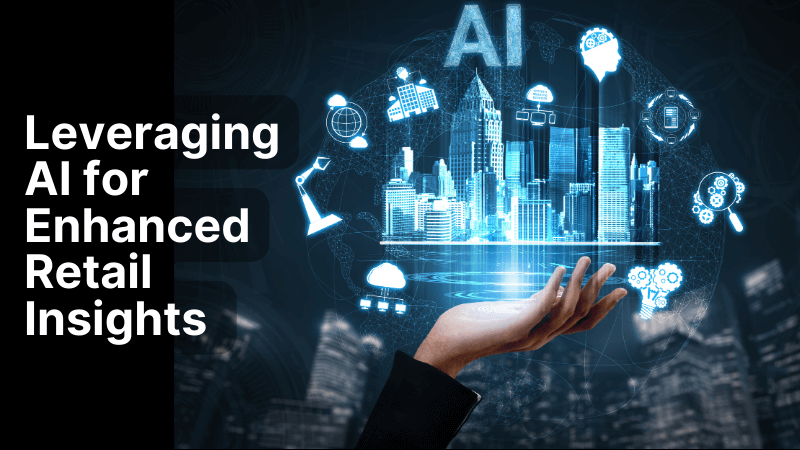
The retail sector stands to benefit immensely from the insights and efficiencies of Artificial Intelligence in analyzing customer reviews. Understanding customer sentiment has become a cornerstone of retail product development, marketing, and customer service strategies. The advent of Large Language Models (LLMs) like OpenAI's GPT-4 and Google's Gemini has revolutionized how businesses harness natural language processing to glean actionable insights from vast amounts of unstructured data.
Following our exploration of Using OpenAI GPT-4 to Perform Sentiment Analysis Data Labeling, this article delves into the application of these groundbreaking technologies within the retail sector, with a particular focus on analyzing product reviews for comprehensive business intelligence.
Following our exploration of Using OpenAI GPT-4 to Perform Sentiment Analysis Data Labeling, this article delves into the application of these groundbreaking technologies within the retail sector, with a particular focus on analyzing product reviews for comprehensive business intelligence.
The Retail Revolution Through Sentiment Analysis
Retailers today face the dual challenge of navigating an increasingly competitive landscape while meeting the heightened expectations of their customers. In this context, product reviews on websites represent a goldmine of customer sentiment, offering unfiltered insights into consumer experiences, preferences, and expectations. Utilizing AI's advanced sentiment analysis capabilities allows retailers to categorize and analyze these reviews at an unprecedented scale and speed, transforming raw data into a strategic asset.
Implementation Examples in Retail
- Product Development and Improvement Models like GPT-4 and Gemini possess a nuanced understanding of language, enabling them to identify key themes and sentiments from product reviews, highlighting areas of strength and opportunities for improvement. For example, a retailer could use AI to analyze customer feedback on a new product line, quickly identifying aspects that resonate well with customers and those that require refinement.
- Personalized Marketing Strategies By analyzing sentiment trends over time, retailers can tailor their marketing strategies to better align with consumer sentiment. This could involve highlighting positively received features in advertising campaigns or addressing concerns raised in negative reviews to demonstrate responsiveness to customer feedback.
- Enhanced Customer Service Sentiment analysis can also prioritize customer service efforts by identifying reviews that express strong negative sentiments, allowing retailers to proactively address issues and mitigate potential negative impacts on brand reputation.
- Competitive Analysis Retailers can extend the application of sentiment analysis beyond their own product reviews to include those of competitors, offering valuable insights into competitive advantages and identifying potential market gaps.
Overcoming Challenges with a Hybrid Approach
Despite the transformative potential of LLMs in analyzing product reviews, retailers must navigate challenges such as data ambiguity and the dynamic nature of language. A hybrid approach, combining the efficiency of the LLM with the nuanced understanding of human annotators, emerges as a robust solution. This strategy ensures the accuracy of sentiment analysis, maintaining relevance and adapting to evolving linguistic trends.
Strategic Advantage in Retail
Incorporating AI into the analysis of product reviews empowers retailers to make informed decisions based on a comprehensive understanding of customer sentiment. This strategic application of AI-driven sentiment analysis positions retailers to enhance product offerings, refine marketing strategies, and improve customer service, ultimately fostering stronger customer relationships and competitive differentiation.
How Shiro Can Help in Retail Sentiment Analysis
The Shiro platform provides a dev environment for prompt engineering and can assist retail businesses in harnessing the full potential of sentiment analysis powered by LLMs like GPT-4, Google Gemini, Mistral, Anthropic, Cohere, or their own fine-tuned models.
Rapid Prompt Engineering Prototyping Shiro provides prompt engineers with an environment to quickly test and iterate on prompt variations, running prompts against a suite of test cases and quantitative evaluation metrics to iteratively measure and improve prompt effectiveness. This capability is crucial for tailoring sentiment analysis prompts to help the AI accurately interpret the wide range of expressions and nuances found in customer feedback.
Retailers can use Shiro to test different prompt strategies against a comprehensive suite of test cases, ensuring the AI effectively captures the subtleties of customer sentiment in product reviews.
Prompt Performance Monitoring With Shiro, retailers can continuously monitor the performance of deployed prompts against quantitative evaluation metrics. This helps teams keep an eye on LLM completion quality over time and monitor for model drift. Prompts that fall out of performance thresholds can then be revised, tested, and redeployed without changing the production code.
Data Collection and Annotation for Fine Tuning The vast and varied nature of product reviews in retail necessitates a nuanced approach to data labeling. Shiro’s capability to store completion enables the collection of explicit feedback on the LLM's performance, either programmatically (via user feedback) or manually (through human review). This feedback becomes a valuable resource for refining the sentiment analysis model, allowing retailers to adjust and their prompting approach based on real customer interactions.
Additionally, Shiro facilitates a hybrid annotation process, wherein LLM-generated labels can be reviewed and corrected by human annotators when necessary. This process not only improves the accuracy of sentiment analysis but also creates a high-quality, proprietary, retailer-specific dataset for custom model fine-tuning.
Rapid Prompt Engineering Prototyping Shiro provides prompt engineers with an environment to quickly test and iterate on prompt variations, running prompts against a suite of test cases and quantitative evaluation metrics to iteratively measure and improve prompt effectiveness. This capability is crucial for tailoring sentiment analysis prompts to help the AI accurately interpret the wide range of expressions and nuances found in customer feedback.
Retailers can use Shiro to test different prompt strategies against a comprehensive suite of test cases, ensuring the AI effectively captures the subtleties of customer sentiment in product reviews.
Prompt Performance Monitoring With Shiro, retailers can continuously monitor the performance of deployed prompts against quantitative evaluation metrics. This helps teams keep an eye on LLM completion quality over time and monitor for model drift. Prompts that fall out of performance thresholds can then be revised, tested, and redeployed without changing the production code.
Data Collection and Annotation for Fine Tuning The vast and varied nature of product reviews in retail necessitates a nuanced approach to data labeling. Shiro’s capability to store completion enables the collection of explicit feedback on the LLM's performance, either programmatically (via user feedback) or manually (through human review). This feedback becomes a valuable resource for refining the sentiment analysis model, allowing retailers to adjust and their prompting approach based on real customer interactions.
Additionally, Shiro facilitates a hybrid annotation process, wherein LLM-generated labels can be reviewed and corrected by human annotators when necessary. This process not only improves the accuracy of sentiment analysis but also creates a high-quality, proprietary, retailer-specific dataset for custom model fine-tuning.
Request a personalized demo to see how retail businesses can use Shiro to implement dependable, adaptive, and accurate AI sentiment analysis as a strategic advantage. Enabling retailers to enhance product development, personalize marketing efforts, improve customer service, and maintain a competitive edge in the marketplace.
The application of Large Language Models like OpenAI's GPT-4 or Google's Gemini for sentiment analysis in retail, specifically through the lens of product reviews, marks a significant opportunity in harnessing AI for business intelligence. By leveraging the unparalleled capabilities of LLMs, retailers can unlock deep insights into customer sentiment, driving strategic decisions that align with consumer needs and preferences. As we continue to explore the vast potential of AI in various business applications, the retail sector stands to benefit immensely from the insights and efficiencies these technologies offer.
For more information, check out this quick overview video:
For more information, check out this quick overview video: